Some features of Bitcoin markets, as summarized above, suggest that market operations and regulatory circumstances that govern markets operations, including the determination of fundamental values, are relevant to both spot and futures prices and would not remain unchanged over time, further suggesting that there might exist dynamic, predictable power from the futures price to the spot, equivalent to a time-varying cointegrating relation.
For example, the sample period of this study covers a dramatic and ultimately volatile booming phase for Bitcoin that was followed by subsequent drops in its value. During this period, the regulatory environments facing the Bitcoin markets saw a series of changes as authorities' regulatory policies on trading Bitcoin underwent a number of adjustments. Mining activities relating to how Bitcoin market operates are also changing over time, contributing to a time variant relationship between Bitcoin spot and futures prices. Examples here include, mining difficulty that adjusts periodically over time, technological changes in computing power to support mining processes, changing energy power for mining, and changing locations for mining activities.
Although time variations limiting arbitrage in the Bitcoin spot and futures markets may be transient, variables of regulatory circumstances and market operations that change over time do not rule out a possibility of a time varying spot-futures cointegrating relation. This paper is devoted to exploring evidence for such relationships. Fourth, the paper also fills a gap by investigating price discovery in Bitcoin spot and futures markets using a time-varying perspective.
The existing literature has found that the ability to assimilate new information in stock indices and foreign currency futures markets in the long run varies over time. These variables are regarded as proxies reflecting non-constant trading over time. In this study we apply concepts from traditional financial assets to the Bitcoin spot and futures markets, while allowing information shares in the Bitcoin spot and futures markets to be time-varying rather than static.
As discussed previously, most of current empirical studies investigate price discovery in the Bitcoin futures markets using the static time-invariant information share methodology.
According to Engle [] , the DCC model can provide a better approximation for the second moment of a multivariate return distribution than other GARCH specifications. Furthermore, we take into account non-zero skewness and excess kurtosis of return distributions in the Bitcoin spot and futures markets for the time-varying price discovery measurements. The findings shed more light on the efficiency of Bitcoin futures markets when market data exhibits asymmetry and fat tails.
Fifth, this paper enriches the literature on the empirical analysis of Bitcoin futures markets by correctly specifying the underlying spot prices for the CBOE and CME futures markets. We suggest that future research uses the correct pair of spot-futures prices for subsequent analysis from a reliable and trusted data source for example, Thomson Reuters Datastream. More specifically, this paper contributes to the literature by highlighting, and demonstrating the effects, of using the correct spot Bitcoin products that underlie the assets specified in the futures contracts traded in the CBOE and CME.
We also emphasize the reliability of databases that contain low-frequency data of the spot assets such as daily data and weekly one. Such information will prove very useful for future researcher on Bitcoin spot and futures prices. With the above contributions, we are able to address the following three important questions from a time-varying perspective:.
Graphical abstract
The remaining parts of the paper are organized as follows. Section 2 describes the existing literature to which we contribute and the particular financial asset issues related to Bitcoin and the testing of causal relationships in this market based upon spot and futures prices. Section 3 discusses the data and presents the econometric methods.
Section 4 presents the empirical findings and Section 5 concludes. Price discovery is generally considered to be an important indicator of the functionality that futures contracts provide towards the underlying spot assets' transactions, and reflects one of the major contributions of futures markets to the organization of economic activity [ Silber, ]. A number of empirical studies support the hypothesis that futures prices absorb new information first, which is then transmitted to the underlying spot market via cross-border transactions etc.
Futures markets, therefore, are generally regarded for most traditional financial assets to lead the underlying spot price in the long run. Much of the theory supporting Granger causality, in this case, is based upon, for example, the assumption that asset prices are driven by the discounted present value of the long-term earnings of shares, or the price dynamics of a bond.
The cost-of-carry model identifies a no-arbitrage condition for futures prices and how a fair price for futures is determined. It allows us to propose that the spot price should not drift away from the futures price for sufficiently long periods given that the no-arbitrage condition ensures that market efficiency is maintained.
The cost-of-carry approach translates into a situation where the long-run equilibrium ties the spot price to the relevant futures price. This theoretical relationship between spot and futures prices translates empirically to a case where estimation and testing can be undertaken within a cointegrating regression framework where the spot and futures prices should be cointegrated as long as the no-arbitrage condition holds. Supporting evidence for such implications have been found widely in the commodity markets and stock index markets see, e. The identification of the price discovery mechanism can be extended to be time variant, since the literature has found that the assimilation of information to reflect intrinsic values can evolve over time.
One of the main consequences is that a time-varying variance and covariance of price innovations occurs, which reflects the evolving process of information generation and assimilation. Attempts to capture the time-varying information share have been recently described in the literature, where there appear to be predominantly three methods established to capture the time-varying nature of price discovery.
How do futures contracts affect Bitcoin prices?
Finally, Avino et al. The informational role of a futures market has been extensively studied by investigating possible lead-lag relationships between spot and futures markets. Granger causality is widely used to formally test for lead-lag relationships temporal ordering to determine which market the spot or futures prices leads the other. Care must be exercised here, especially the need for robustness of the results, as it is well-documented that Granger causality tests can be very sensitive to the time period of estimation or to assumptions that causal relationships do not change time invariant over time.
The procedure of Shi et al. In addition, the nature of the cointegrating relationship between spot and futures prices has important implications. For futures and spot markets there exist a priori expectations that there is a strong cointegrating relationship between the two markets. If spot and futures prices are cointegrated, spot-futures parity exists, indicating that no arbitrage opportunities arise.
Moreover, the presence of cointegration also shows that futures markets are efficient.
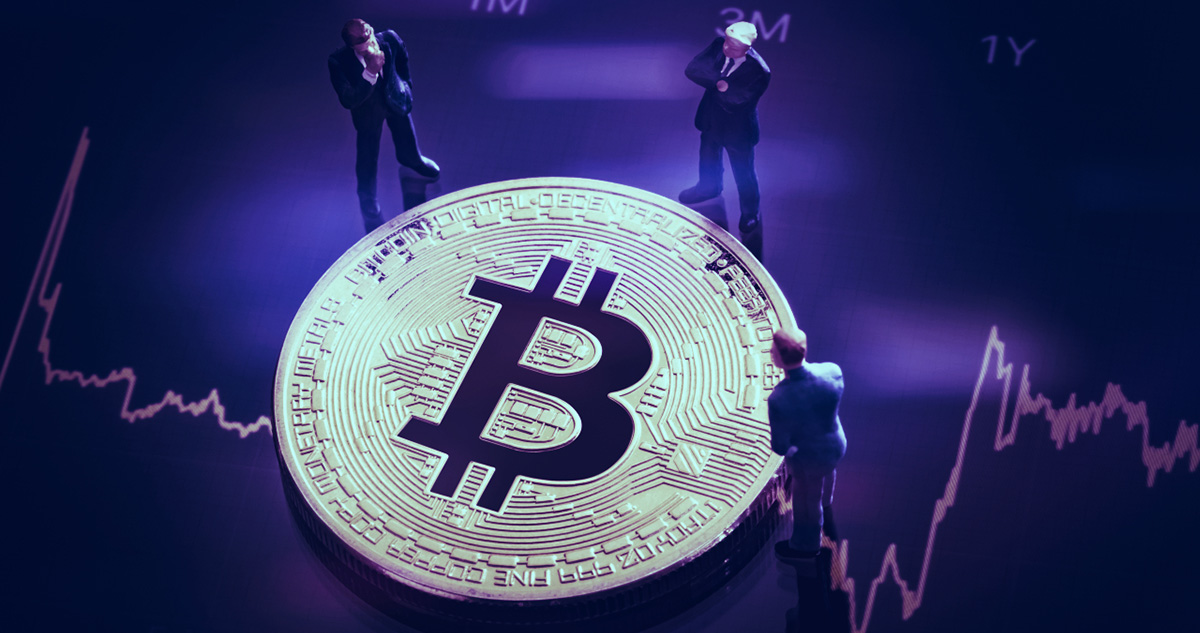
However, when testing for such conditions, conventional cointegration tests assume a static time-invariant framework. If this assumption is invalid, cointegration may be falsely rejected as well the implications listed above, hence, it is important to allow for a time-varying cointegration framework where time invariance is a special case which enriches the potential interactions between variables when they are driven by the same information set.
The advent of cryptocurrency trading has led to considerable discussion about what determines their prices and in particular the links between spot and futures prices. As discussed above, grounding cryptocurrency pricing within a cost-of-carry framework embeds the no-arbitrage condition into the pricing process, which empirically translates into the existence of a cointegrating relationship framework.
Bitcoin Faces Volatility Rise as Futures Market Shows Signs of Overheating - CoinDesk
Booth, So, and Tse [] , Jong and Donders [] , Fleming, Ostdiek, and Whaley [] and Hsieh, Lee, and Yuan [] argue that, in a trading cost world, futures trades have a lower trading cost and faster information absorption. The theoretical rationale of futures' superiority in information content has also been well discussed and explained by Fleming et al. However, this work pre-dates the emergence and growth of cryptocurrencies and the massive growth of new information including from e.
Cryptocurrencies are so different to traditional markets that using the risk-free rate as a key compounding factor may not be appropriate and the key concept of risk neutrality has yet to be fully investigated and tested in this type of market. As such, the question as to whether the cost-of-carry approach is an appropriate one for pricing cryptocurrency-based futures has not been established, but can be addressed via various tests including cointegration, lead-lag causality and price discovery stability.
Given some of the unique features of cryptocurrency spot and futures prices summarized in the Introduction, one might expect some differences in the cryptocurrency futures pricing model compared to other asset markets. Place this in a world of disruption coming from e. The nature of the data used and econometric flexibilities employed are discussed in Section 3 below.
- fork attack bitcoin;
- er bitcoin sikkert.
- Bitcoin futures launch to test Ether’s spectacular rise.
- price chart of bitcoin in usd.
- brick bitcoin.
- Subscribe to read | Financial Times;
- buy bitcoins immediately?
BRR refers to the daily reference rate of the U. The BRR aggregates the trade flows from the major Bitcoin spot exchanges, for example, Bitstamp, Coinbase, itBit and Kraken at 4 pm London time to ensure transparency and replicability in the underlying spot markets. We switch the most nearby contract to the second most nearby one if the trading volume of the former is exceeded by the latter at the former's contract month. The Gemini auction price and the BRR are used to represent the spot markets under consideration in this paper. After matching the spot and futures data series, we are left with observations for the CME sample and observations for the CBOE sample.
Donate to arXiv
Note that our sample excludes data on weekends due to unavailability. Both the spot and future prices are downloaded from Thomson Reuters Datastream for our empirical analysis. It should be noted that the futures and spot prices used by the CBOE and CME are different, hence the empirical analysis is based on the counterpart spot markets. All spot and futures prices are transformed to natural logarithms and are presented as Fig. As can be seen from the figure, there is a decreasing trend for both spot and futures prices from the beginning of the sample period until the early of February , which might represent a bear market in both the spot and futures markets.
From early February , both prices follow an upward trend until the end of sample period, which suggests a bull market. Second, the patterns of both spot and futures prices look similar. It is possible that there exists a long run relationship between spot and futures prices. This will be further examined formally via tests for cointegration. We also provide descriptive statistics on Bitcoin spot and futures daily returns in Table 2. As can be seen from the table, the means of spot and futures returns are negative.
The volatilities of the four markets are similar. Second, returns of the four markets do not follow a normal distribution as indicated by a Jarque-Bera test. This might be due to non-zero skewness and excess kurtosis, which will be further examined via a semi-nonparametric SNP approach. Finally, heteroscedasticity may exist in the spot and futures returns given the existence of significant Ljung-Box Q statistics. The volume of CBOE Bitcoin futures dominates in the early markets from December to the middle of after which the CME's product starts to dominate in the market and this phenomenon becomes more evident when the CBOE decided to stop listing its product in March Thus it is interesting to investigate the pricing behaviour of futures contracts in these two markets resulting from those transactions.
Daily returns are calculated as the first order differences of log daily prices. JB , the Jarque-Bera test statistic for normality. LB 2 12 denote the Ljung-Box Q test statistic for return squares up to lag order The following section is taken from Shi et al. We can write an unrestricted VAR p in multi-variate regression format simply as:.
In order to test the null hypothesis that y 2 t does not Granger cause y 1 t , the Wald test for such restrictions can be denoted as:. Each row picks one of the coefficients to set to zero under the non-causal null hypothesis. There are p coefficients on the lagged values of y 2 t in Eq.
Following the recent bubble detection tests of Phillips, Shi, and Yu [] , Shi et al. If the Wald statistic sequence exceeds its corresponding critical value, a significant change in causality is detected.

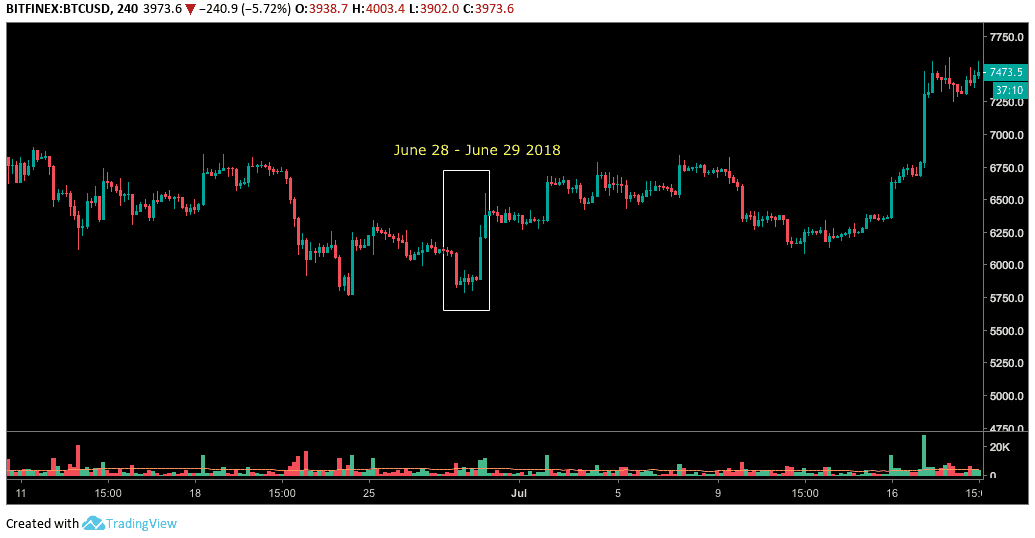
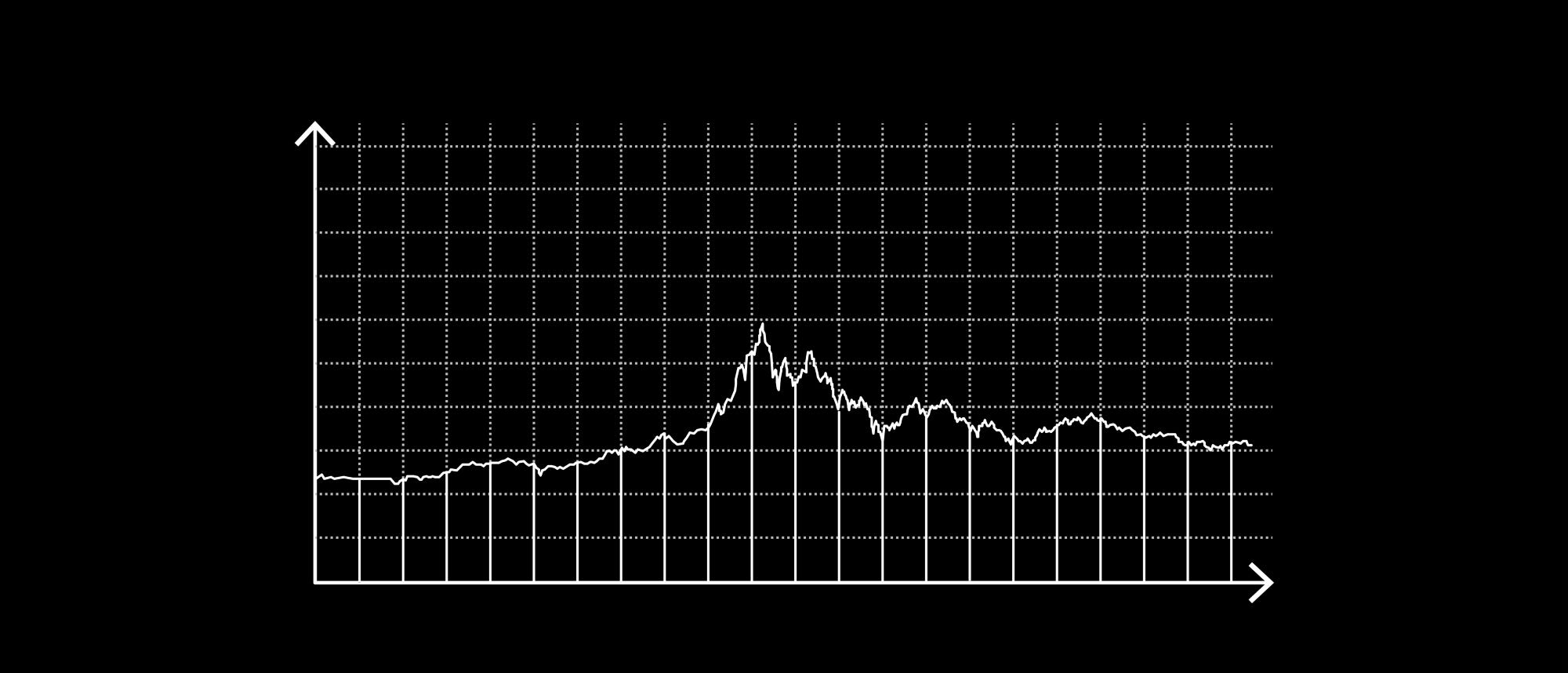

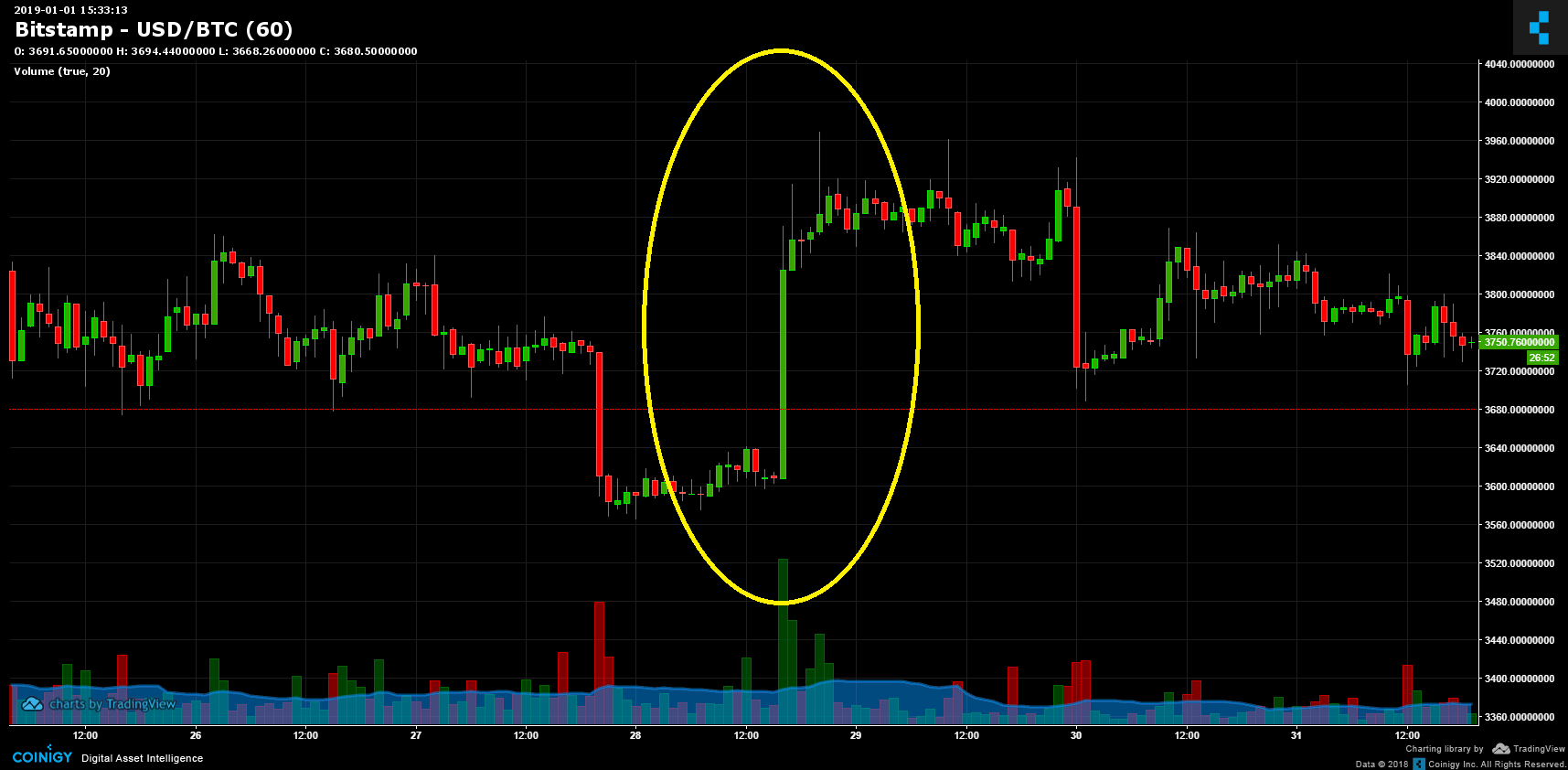
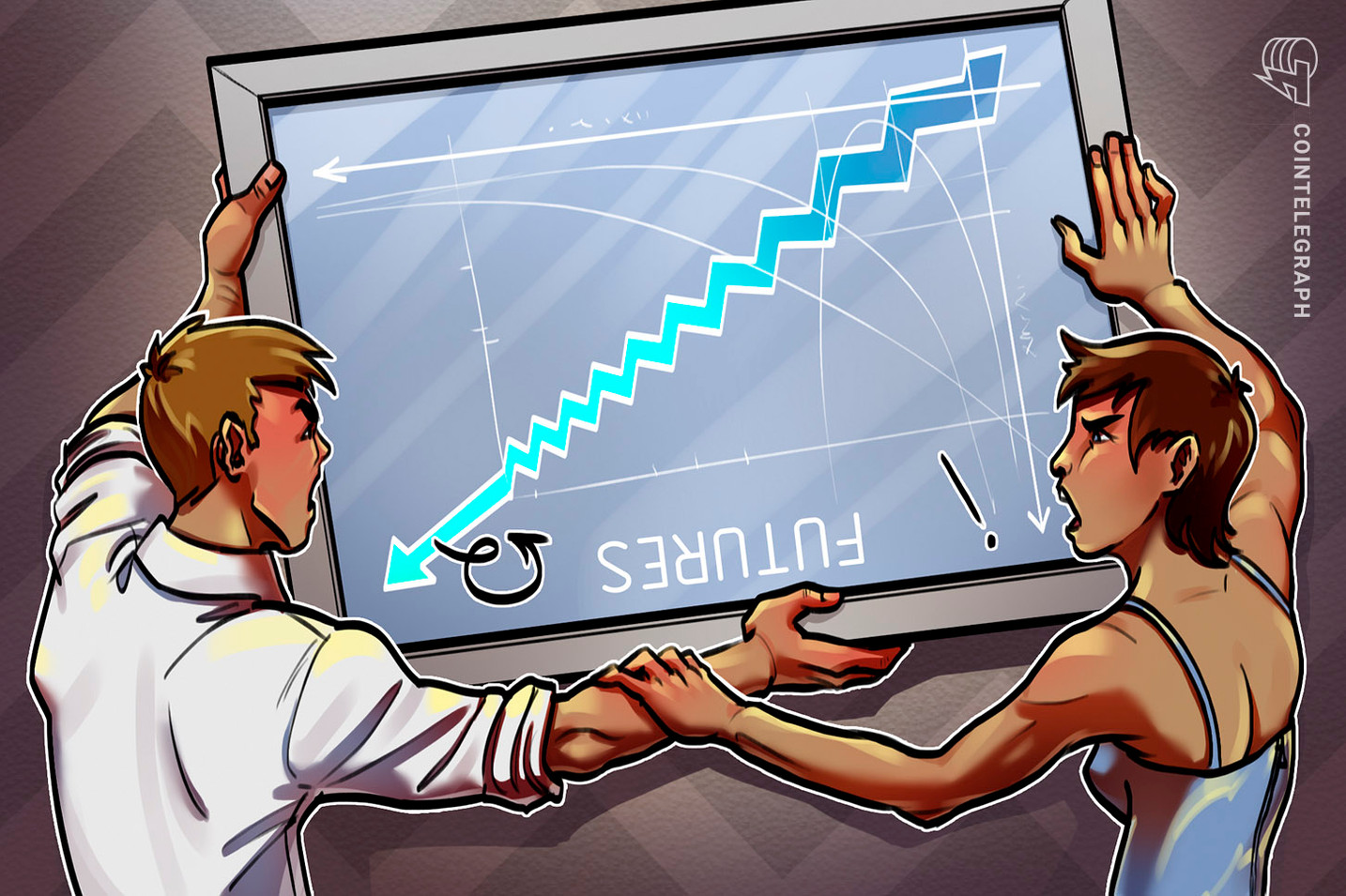

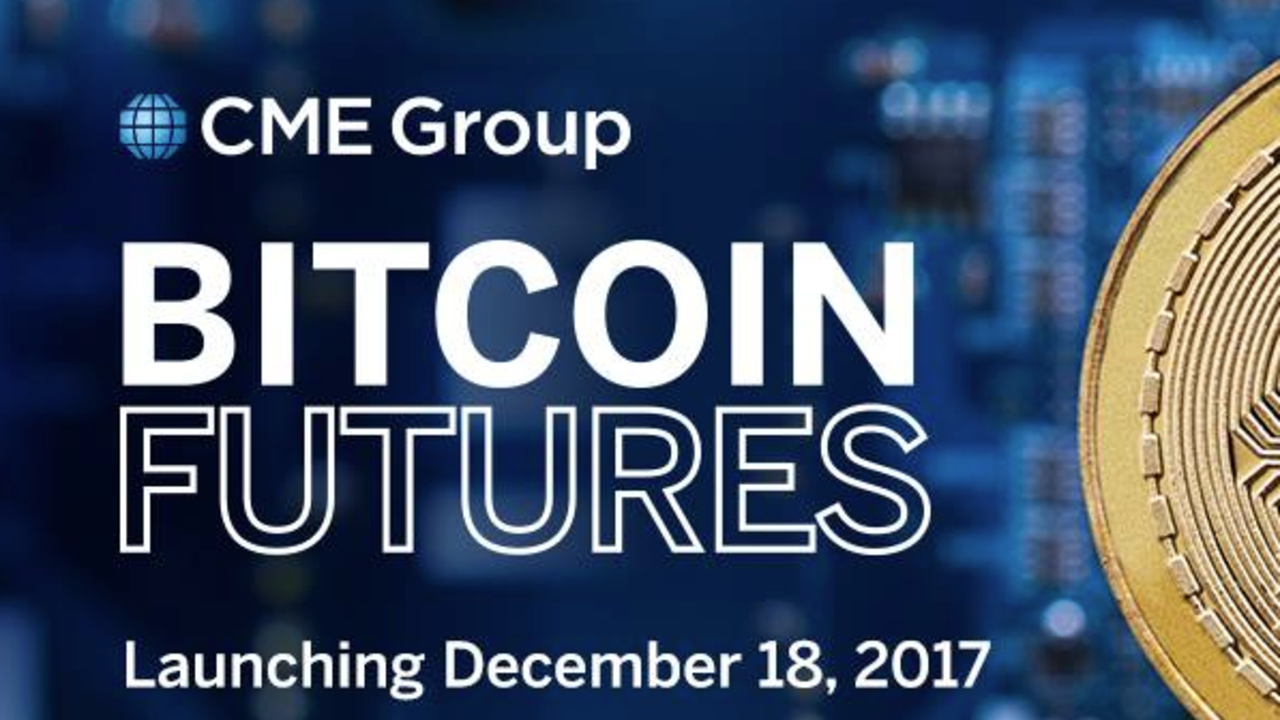
Related the effect of futures on bitcoin
Copyright 2020 - All Right Reserved