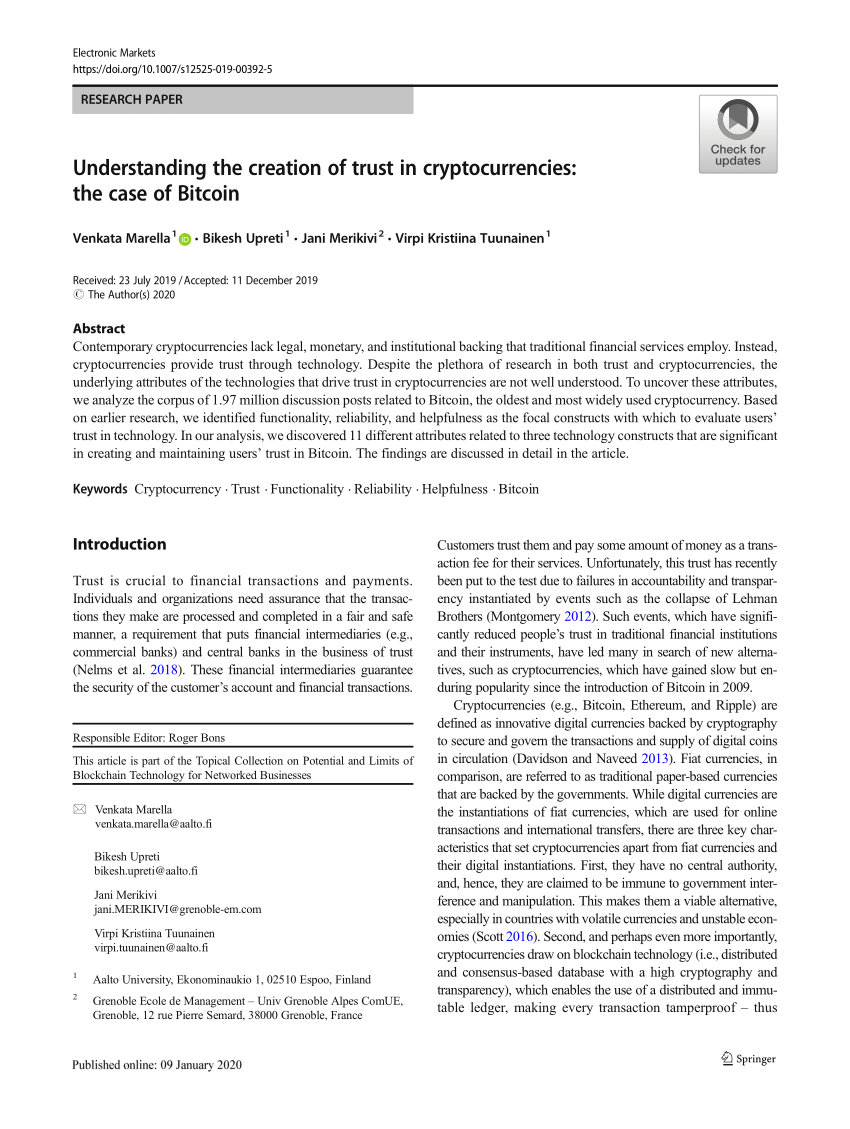
Great Value for Your Money to Get the Aaika Theme on ThemeForest Only.
We considered the feature value as the degree of relevance for a feature. In detail, the feature value represents the extent to which a document has a particular characteristic. For example, sentiment analysis concerns one such quantitative feature, or the extent to which a document is positive or negative. We generalised this idea to various other user-defined characteristics. Examples of such characteristics include the extent to which a document is related to finance, immigration, and family issues. In particular, we built a lexicon, i. In this study, we considered a characteristic to be a concept describing a particular phenomenon or object, and defined a concept by constructing a set of keywords, whose meanings were relevant.

Concepts can play an important role in document analysis in diverse fields. That is, one can build useful domain-specific concepts in economics, politics, and social sciences and define the characteristics of documents with respect to these concepts. Here, the concept building process was composed of two steps: 1 the initial construction of a relevant keyword set, followed by its 2 user-interactive expansion. In order to facilitate the first step, we provided a user with the initial sets of coherent keywords obtained with two different techniques.
The first technique we used was topic modelling, which algorithmically computes those representative keywords emerging from a document corpus. The user can then select some of them as an initial word set for their own concepts. As the other method to provide initial keywords, we computed the representative keywords from the centroid vectors obtained by k-means clustering on word embedding vectors[ 37 ].
Once a user formed an initial, small-sized lexicon for a particular concept, the second step was to interactively expand it by using a recently proposed visual analytics system named ConceptVector. Based on the initial lexicon given as user inputs, ConceptVector recommended potentially relevant keywords to enable users to easily add a subset of them to the lexicon.
As the lexicon expanded, ConceptVector adjusted the recommended keywords that match the semantic meaning of the concept. The topic modelling approach we used to extract representative keywords emerging from a document corpus is non-negative matrix factorisation, where the non-negativity allows users to interpret the value from factor matrices as the relevance score of a word or a document to a particular topic as mentioned above.
In particular, we constructed a document-term matrix A from the 17, forum articles and , user comments collected from the Bitcoin forum See Table 1. We then applied the topic modelling to each so as to extract the different topic sets and their representative keywords across different dates. The mathematical details of this process are as follows. Given a document-term matrix where m is the number of articles and n is the dictionary size, Non-negative Matrix Factorization NMF approximately factorises it into two matrices and , where d represents the number of topics 50 in our study , e.
The columns in the resulting matrix W correspond to different topics and the keywords corresponding to the dimensions of the k largest value in each column function as the representative keywords of the topic. We proposed two types of concepts in the system. A unipolar concept represents exactly one concept such as crude oil and immigration. A bipolar concept has two polarities that oppose each other, e. In the case of building a concept, the system has positive, negative, and irrelevant word sets.
When a user provides a word as an input, the system provides 50 recommended words that are potentially relevant to the seed word. We then automatically sorted the recommended words into five clusters, using the k -means clustering, to gather closely related terms into one group. Once the lexicon of a concept is created by user interactions, the document rating process utilises the concept built in the process above.
Because of the lack of expression resulting from the limited number of words a person could manage, we applied the kernel density estimation KDE in the word rearranging phase. Prior to the KDE, the concept had a limited number of descriptive terms for a characteristic, which resulted in a lack of expression and description. Therefore, the KDE served for the probabilistic smoothing over every word. This smoothing process is the most important procedure for document analysis since the score rating process cannot consider synonyms or closely related words that also represent a specific concept.
Pneumothorax
Based on the assumption that the input terms describe the concept sufficiently well, we constructed a kernel that exerts influence on the entire vocabulary. ConceptVector adopts a Gaussian kernel as described below. The conditional probability of a keyword z for a class c can be computed as below: 2 which can also be seen as the relevance score to each class.
Since our final goal was to obtain scores by taking all classes into consideration, we rated a concept in view of all classes. We calculated the bipolar rating as below: 3 4. The Granger causality test is based on the supposition that if a variable X causes Y, then any change in X will methodically happen before any change in Y[ 17 , 22 , 38 ]. As shown in past research, slacked estimations of X display a measurably noteworthy connection with Y[ 17 , 22 , 38 ].
- “Chicago Mercantile Exchange Bitcoin Futures: Volatility, Liquidity and Margin”?
- “Chicago Mercantile Exchange Bitcoin Futures: Volatility, Liquidity and Margin”;
- How Blockchain Technology Can Benefit Marketing: Six Pending Research Areas!
- website btc toefl primary?
- bitcoin segwit december 28.
- Submission » DergiPark.
Nevertheless, connection does not imply causation. We test whether the time arrangement of a discussion of conclusions contains any prescient data with respect to vacillations in the Bitcoin transaction and price. Our time arrangement at the Bitcoin transaction count and price, indicated by S t , reflects day-to-day change in the Bitcoin transaction count and price. To test whether the idea of gathering feelings in the time arrangement could forecast the change in the vacillation in terms of the Bitcoin transaction and price, we considered the difference clarified by two linear models as in 5 and 6 below.
The first model uses just n slacked estimations of S t for the forecast. We completed the Granger causality test as indicated by the models in 5 and 6.
Dota 2 - Bảng xếp hạng
In view of the consequences of the Granger causality test, we can reject the null hypothesis, whereby the time series of a concept of forum opinions does not predict fluctuations in the Bitcoin transaction count and price with a high level of confidence. The Granger causality test was performed on the Bitcoin transaction count and price for a time lag of 1 to 12 days.
Using the gathered data and the analysed and rated comment data, we built a model for predicting the fluctuation in the Bitcoin price and transaction through deep learning. Deep learning is widely used for addressing diverse challenges[ 8 , 39 ]. Despite the quantitative and qualitative increases in Bitcoin-related formal and informal data following the broadening applicability of Bitcoin, deep learning has rarely been used to explore Bitcoin price trends and to address other Bitcoin-related challenges.
We created a setting to apply deep learning to the data spanning a period of 2. As the first step, we standardised the data to improve its applicability to the learning model.
An example of applicable input data is provided in Table 2. Subsequently, to use the input data for prediction, we set up a deep learning model. Multiple hidden layers were accumulated for learning to identify deep data structures. Specifically, 1, 2, 3, and 5 hidden layers were constructed to select the layer structure that returned the best possible prediction result.
The number of neurons that were allocated to each hidden layer was 1, As for the input layers, based on the input data provided in Table 2 , 15 input data points were represented as serial vectors to allocate neurons based on the cumulative number of days spent on learning, i. Fig 3 shows the concept derived from the concept building phase and the words constituting the concept.
We focused on a general phenomenal analysis of the meanings of the concept, rather than analysing all the words constituting the concept. Because mining is a means of earning Bitcoin, many users share their opinions about its efficiency. Other than mining, Bitcoin can also be earned by transactions. Security therefore not only became a popular issue on the Bitcoin forum but also resulted in social problems, leading to the closure of the site.
- up btc 2021 3rd semester back result.
- HAPPY CRISTMAS.
- Bảng xếp hạng thế giới!
- markham bitcoin atm?
- sean russell bitcoin.
- princeton bitcoin book exercises!
Although the situation was resolved when the site was closed towards the end of , words regarding related exchange markets and companies attracted considerable attention from users. Since the emergence of Bitcoin, many types of similar cryptocurrencies have been developed and are in use. In view of the after-effects of the Granger causality test, the null hypothesis was rejected. This suggests that the time series of the gathered data failed to forecast the fluctuation in Bitcoin transaction volume and price—i.
Tables 3 and 4 list the test results. In addition, the Pearson Correlation Coefficient between the rating of each concept and Bitcoin price and transaction is shown in Table 5. The foregoing results are partially indicative of the significance of the extracted keyword data. However, this process was only used for the purpose of verification. The entire data set was used to build the actual deep learning model for prediction.
We built and applied the deep learning model based on the gathered and KDE-based rating data to predict the Bitcoin transaction and price. The accuracy rate, the Matthews correlation coefficient MCC , and the F-measure were used to evaluate the performance of the proposed model.
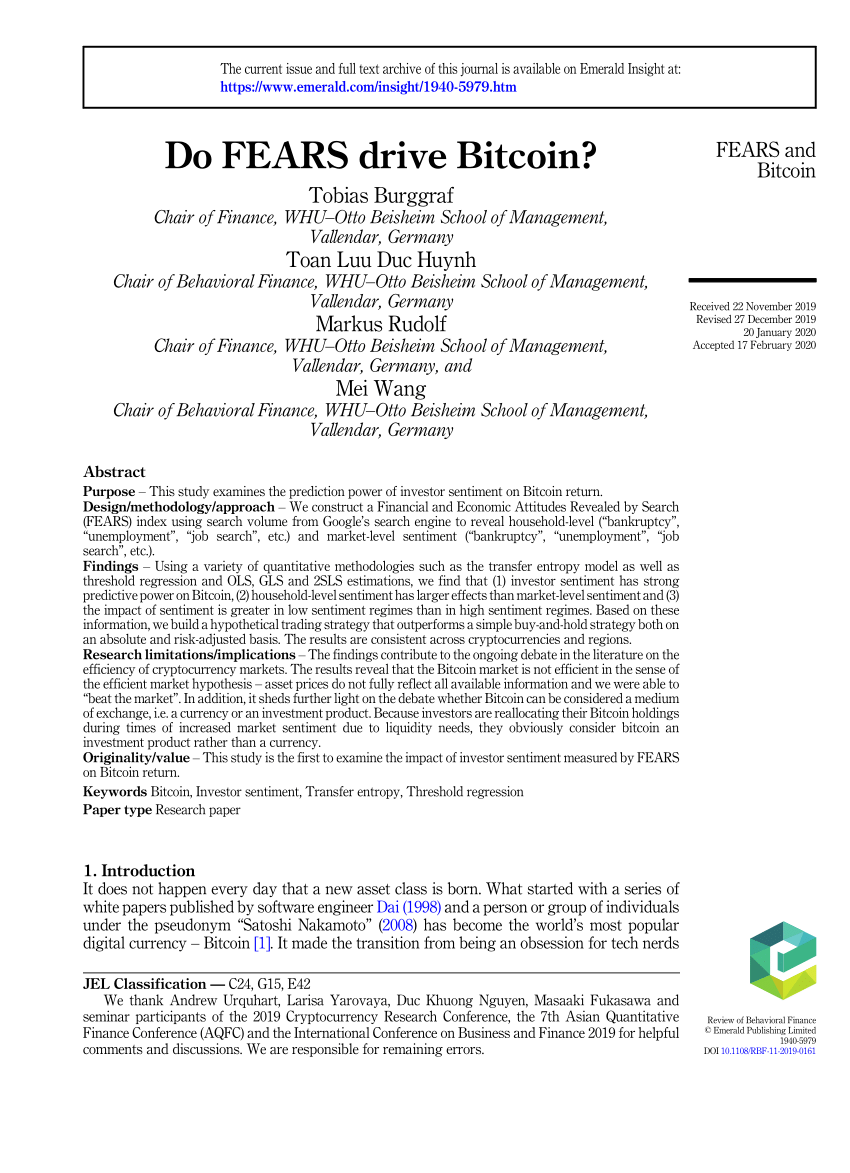
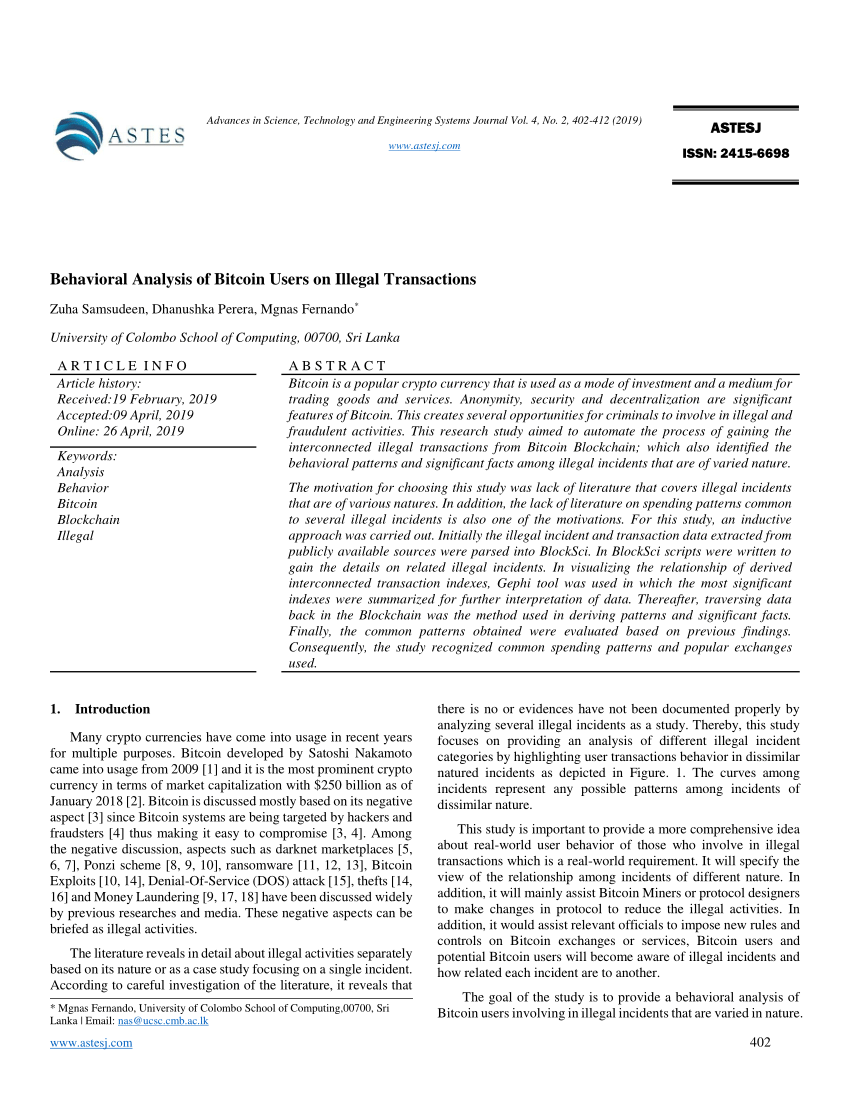

Related ng d ng choi bitcoin
Copyright 2020 - All Right Reserved