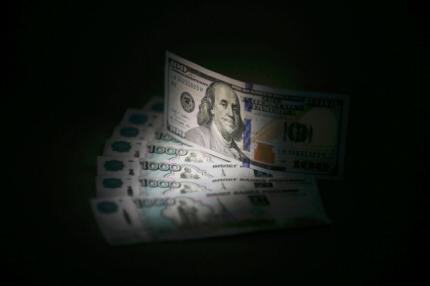
A new unapproved transaction is called a Tip. Each new Tip should reference two previous transactions. Ideally, the network uses the Random Walk Monte Carlo algorithm to ensure that only good tips are chosen. This consensus method is effective because every new tip must approve two older tips before it can be considered valid itself. This means that as more devices are added to the network it theoretically becomes easier to find consensus.
The problem with this method, however, is that it requires a very large network to function correctly. So initially, all Tip validation is controlled by coordinator nodes. Every minute the coordinator node makes a normal transaction with its signature on it, known as a milestone. In order for a transaction to be considered verified, the newest milestone must directly or indirectly verify your Tip.
A milestone signature cannot be faked, so this helps prevent a malicious actor from hijacking the Tangle and creating fake signatures. While an effective solution, the coordinator node has received a great deal of criticism for being too centralized.
IOTA BTC (MIOTA-BTC)
The argument is that if the coordinator node is somehow compromised then it could create two separate Tangles and double-spend funds on the network. IOTA has argued that while the attack is theoretically possible, it would be economically illogical, so should not be a concern. They have also stated that the Coordinator node is a purely temporary solution to the small network size. Once the network is large enough to be self-sustaining, the Coordinator will be turned off and the Random Walk Monte Carlo algorithm will be used in its place. Despite being a unique currency, the price of IOTA is governed by the same factors as more traditional blockchain-based cryptocurrencies.
Even by cryptocurrency standards, the price of IOTA has always fluctuated wildly. One of the most important price drivers for a service-focused cryptocurrency, like IOTA, is corporate partnerships. Media attention and hype about new features also play a key role in determining the price of a cryptocurrency. If a token appears in the news regularly, you should expect to see a sudden surge in price. This is often followed by a short, sharp corrective period as traders engage in profit-taking. If IoT devices continue to grow in popularity, IOTA will serve an incredibly useful purpose by facilitating simple machine-to-machine transactions.
MIT media lab quickly shot back, arguing that many of the points in the technology review article were either incorrect or misleading.
IOTA (MIOTA) Live Price, Chart, Volume, Supply, Market Cap & Overview
It is notoriously difficult to design your own cryptography and most systems undergo years of rigorous tests before they are allowed to go live. When told about the vulnerability, Bruce Schneier, a renowned technology and security expert, said:. In , leaving your crypto algorithm vulnerable to differential cryptanalysis is a rookie mistake. It says that no one of any caliber analyzed their system and that the odds that their fix makes the system secure is low.
Remembering how quickly Nxt protection was disarmed I was keeping in secret the fact of the existence of such mechanism in IOTA. I was pretty sure that the protection would last a long time because it was hidden inside the cryptographical part and programming skills would be insufficient to disarm the mechanism.
Many, such as Ethereum developer, Nick Johnson, have argued that this excuse is not only inadequate but even hostile towards the very concept of open source technology:. Despite the open hostility of many experts and developers from other cryptocurrencies , IOTA has found support from other sectors. A large number of companies have been working with IOTA to attempt to develop their data marketplace. See our full guide to trading IOTA , or start your research with reviews of these regulated crypto brokers available in.
CFDs are complex instruments and come with a high risk of losing money rapidly due to leverage. Please Note: Availability subject to regulations. Skip to content. Disclosure: Your support helps keep Commodity. Learn more The price of Bitcoin in USD has considerably increased in the period considered. Note that, in Figure 16 , we have made predictions and computed portfolios considering prices in Bitcoin. Then, gains have been converted to USD without transaction fees.
In Table 2 , we show instead the gains obtained running predictions considering directly all prices in USD. We find that, in most cases, better results are obtained from prices in BTC. In Figure 17 , we show the geometric mean return obtained by between two arbitrary points in time under geometric mean return optimisation for the baseline Figure 17 a , Method 1 Figure 17 b , Method 2 Figure 17 c , and Method 3 Figure 17 d.
IOTA Price Prediction 2021 And Beyond: What to Expect?
The data used to support the findings of this study are available from the corresponding author upon request. This is an open access article distributed under the Creative Commons Attribution License , which permits unrestricted use, distribution, and reproduction in any medium, provided the original work is properly cited. Journal overview. Special Issues. Academic Editor: Massimiliano Zanin.
Received 29 May Revised 28 Sep Accepted 17 Oct Published 04 Nov Abstract Machine learning and AI-assisted trading have attracted growing interest for the past few years. Materials and Methods 2. Data Description and Preprocessing Cryptocurrency data was extracted from the website Coin Market Cap [ 61 ], collecting daily data from exchange markets platforms starting in the period between November 11, , and April 24, Figure 1.
Number of cryptocurrencies. The cryptocurrencies with volume higher than as a function of time, for different values of. For visualization purposes, curves are averaged over a rolling window of days. Figure 2. Return on investment over time. The daily return on investment for Bitcoin orange line and the average for currencies with volume larger than USD blue line. Their average value across time dashed lines is larger than. Figure 3. Schematic description of Method 1. The training set is composed of features and target T pairs, where features are various characteristics of a currency , computed across the days preceding time and the target is the price of at.
The features-target pairs are computed for all currencies and all values of included between and. The test set includes features-target pairs for all currencies with trading volume larger than USD at , where the target is the price at time and features are computed in the days preceding. Figure 4. Schematic description of Method 2. The training set is composed of features and target T pairs, where features are various characteristics of all currencies, computed across the days preceding time and the target is the price of at. The features-target pairs include a single currency , for all values of included between and.
The test set contains a single features-target pair: the characteristics of all currencies, computed across the days preceding time and the price of at. Figure 5. Cumulative returns.
The cumulative returns obtained under the Sharpe ratio optimisation a and the geometric mean optimisation b for the baseline blue line , Method 1 orange line , Method 2 green line , and Method 3 red line. Analyses are performed considering prices in BTC. Figure 6. Geometric mean return obtained within different periods of time. Note that, for visualization purposes, the figure shows the translated geometric mean return G Shades of red refer to negative returns and shades of blue to positive ones see colour bar.
Figure 7. Feature importance for Methods 1 and 2. Results are shown for and. Results are shown for , , for Ethereum b and Ripple c. For visualization purposes, we show only the top features.
Figure 8. Baseline strategy: parameters optimisation. The sliding window a, c and the number of currencies b, d chosen over time under the geometric mean a, b and the Sharpe ratio optimisation c, d. Figure 9. Method 1: parameters optimisation. The sliding window a, d , the training window b, e , and the number of currencies c, f chosen over time under the geometric mean a, b, c and the Sharpe ratio optimisation d, e, f.
Figure Method 2: parameters optimisation.
IOTA Chart
Method 3: parameters optimisation. The median squared error of the ROI as a function of the window size a , the number of epochs b , and the number of neurons c. Results are shown considering prices in Bitcoin. The number of currencies chosen over time under the geometric mean a and the Sharpe ratio optimisation b. Upper bound for the cumulative return. The cumulative return obtained by investing every day in the currency with highest return on the following day black line.
The cumulative return obtained with the baseline blue line , Method 1 orange line , Method 2 green line , and Method 3 red line.
Results are shown in Bitcoin.
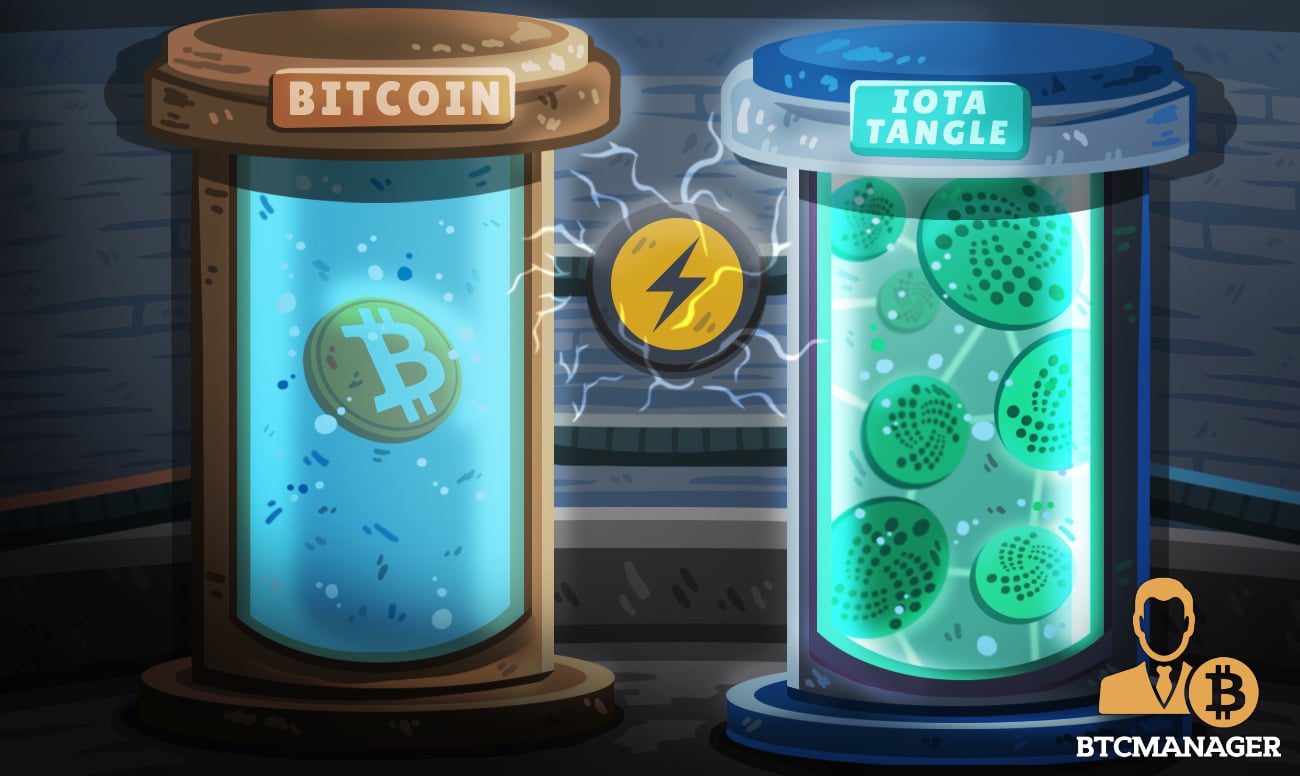

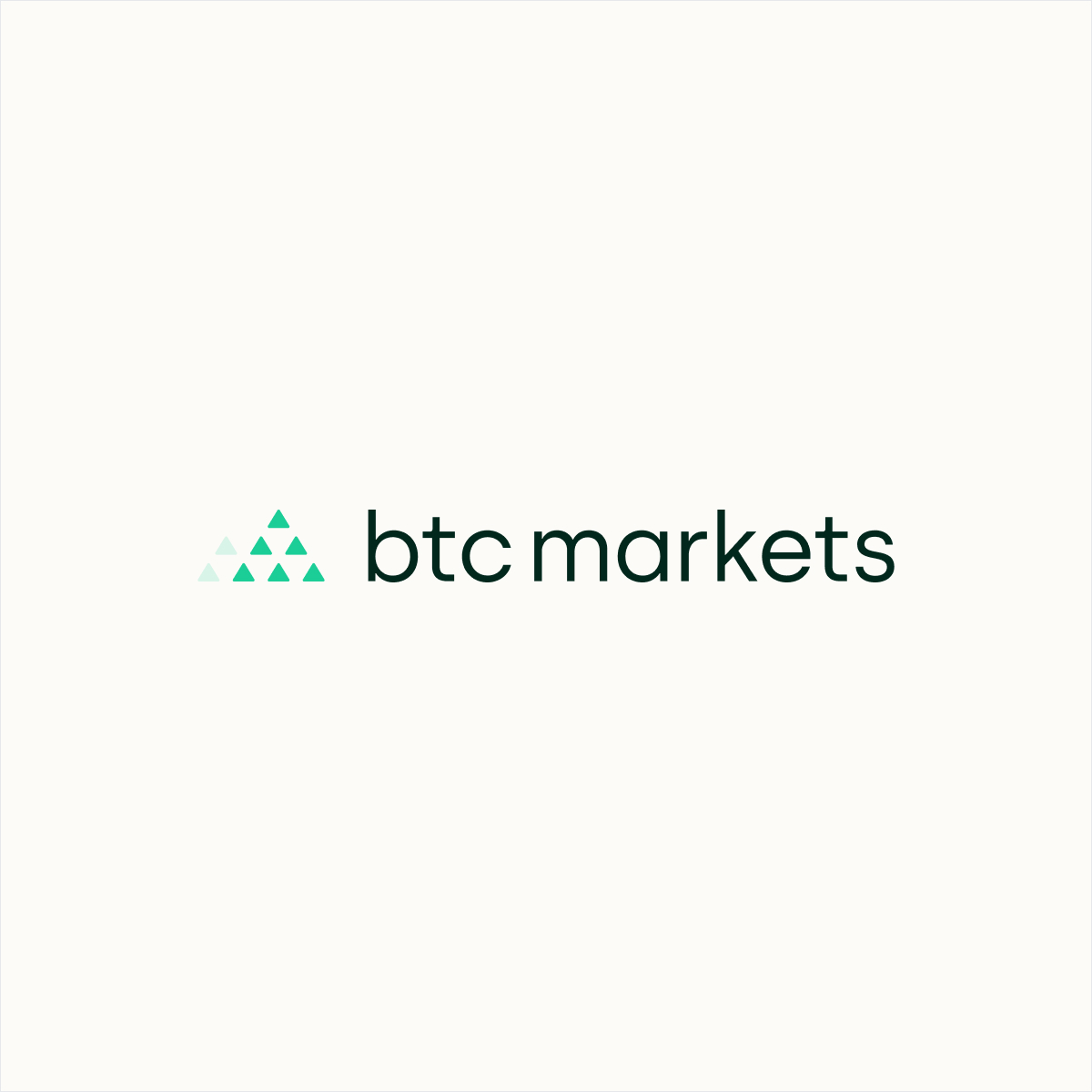

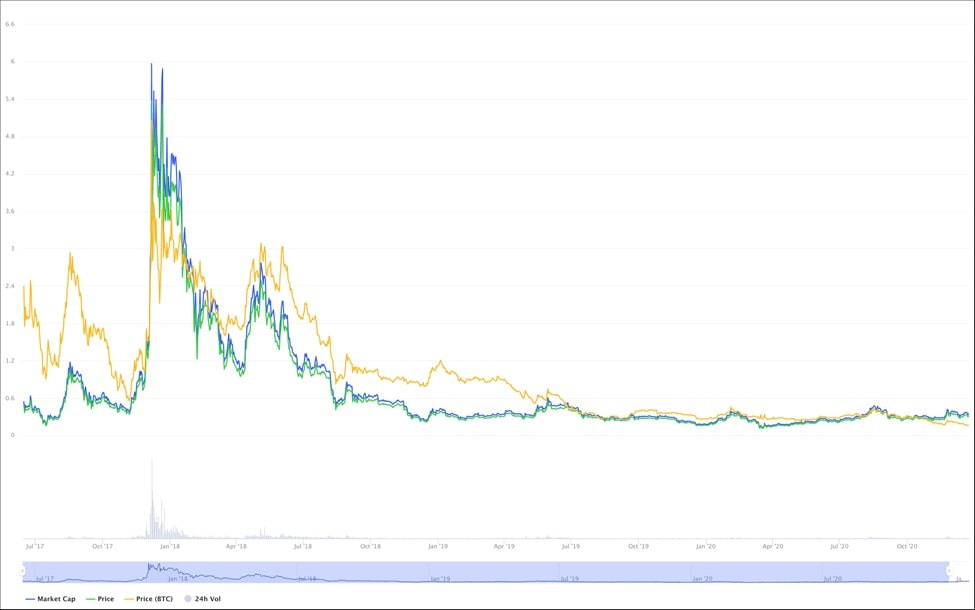
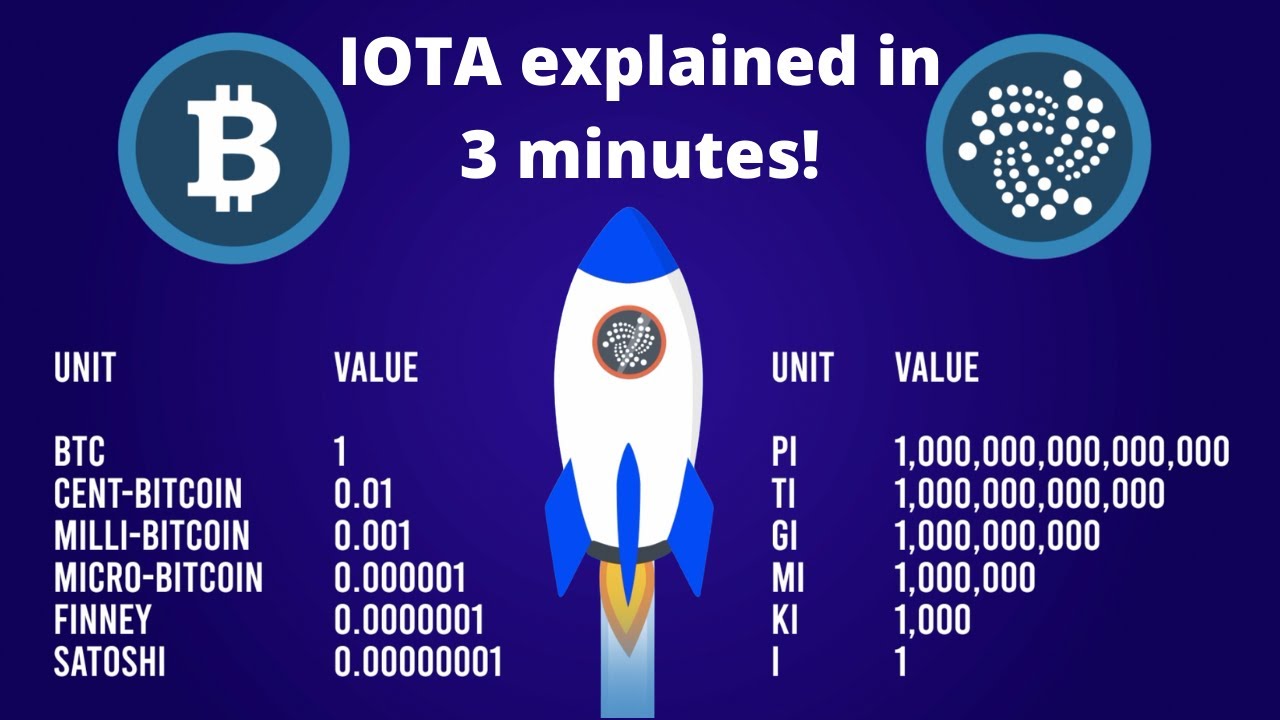

Related btc markets iota
Copyright 2020 - All Right Reserved