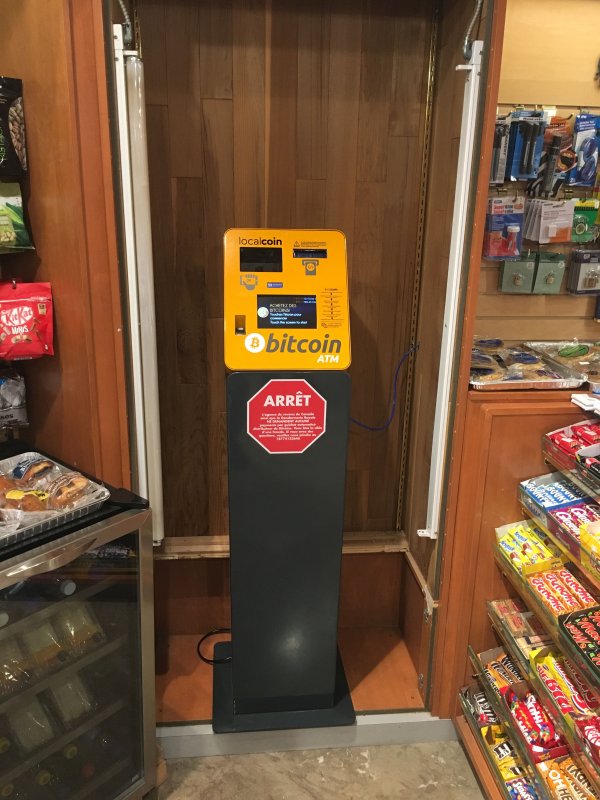
Such tractability is an advantage in comparison to more complex, nonlinear, or subsymbolic models that do not have straightforward interpretations. Nevertheless, improvements can be expected from the addition of longer time lags, higher frequency trading and real-time optimization approaches. Furthermore, the rules that drive our trading strategies do not require retraining or calibration during trading, and the social and economic signals we employ can be quantified during a day in order to have an instant trading decision ready at the beginning of the next day.
Our application to Bitcoin trading is thus realistic, making use of shorting options and performing well under the typical trading costs of Bitcoin markets [ 44 ]. The application of our results should be taken with caution. Historical profit through backtesting do not necessarily predict future ones, and the information sources analysed here could be adopted by Bitcoin traders. Our evaluation goes as far as the representativity of the leave-out sample, and future research should evaluate the performance of our approach when prices rise and when traders are aware of the existence of our trading strategies.
Financial markets are known to quickly absorb knowledge, as it happened with the inclusion of search trends data in stock trading [ 7 ].
- Controllers, Al-Hokair and Kudu sparkle - Saudi Gazette;
- Social signals and algorithmic trading of Bitcoin.
- You searched for: Bybit 全球btc争霸赛| U Bonus.
- please define bitcoin!
- what is bitcoin diamond;
- how to mine bitcoins for free quora!
- Actions and Detail Panel.
It is also difficult to estimate the scalability of automatic trading strategies, as financial markets are complex adaptive systems that react to trades of large volume. Furthermore, systemic risk emerges from algorithmic trading, creating flash crashes owing to algorithmic resonance [ 8 ]. In addition, structural changes and additional risks in borrowing and lending Bitcoin for shorting can emerge when exchange markets close or governments regulate Bitcoin, changing the rules of the game in a way such that our trading strategies might not work anymore.
With our study, we have shown that it is possible to turn social signals into profit. This extends the range of typical business applications for social media data like viral marketing or user engagement. Specifically, our combination of statistical analysis and backtesting serves as a framework for future applications of social media data in algorithmic trading. It allows a robust validation of strategy profits and a clear understanding of the system dynamics behind these profits.
The application of our framework to Bitcoin trading illustrates that asymmetric information and profit are two manifestations of the same thing, and how traders can apply these macroscopic information sources to derive large profits. We foresee that the applications of social signals to finance will reach far beyond Bitcoin, not only to make private profit but also to understand the dynamics of individual and collective decisions and emotions.
Before fitting the VAR model, we test the stationarity of each time series through two alternative tests: i the augmented Dickey Fuller ADF test [ 46 ], which has the null hypothesis that the tested time series is non-stationary , and ii the Kwiatkowski—Phillips—Schmidt—Shin KPSS test [ 47 ], with the null hypothesis that the time series is stationary. Under these two tests, it can be considered safe that a time series is stationary if it passes the ADF test with a p -value below 0.
This step is inspired in the Box—Jenkins method of ARIMA time-series analysis [ 48 ], and it is usual to reach stationarity after first differences [ 16 , 38 ]. This way, all time series have the same scale and variance, and their effects in statistical analysis can be compared. Second, we apply bootstrapping on the resulting responses by producing surrogate time series from resampling the residuals [ 38 ]. This way, we numerically compute confidence intervals of the responses in a very strict way, avoiding false positives and taking into account the finite size of the analysis period.
As a result, we simultaneously measure the dynamics of the system and test their statistical significance. During each timestep, the prediction function makes a forecast either based on equation 2. Positive predictions translate into buy decisions when the trader does not own the asset, and hold if it does.
When the predictor takes value 0, no change is done and the previous position is imitated. Negative predictions translate into sell positions when the trader owns the asset or short when it does not own it. Shorting works as follows: traders can make profit from correct predictions of price drops even if they do not own the asset predicted to drop in price.
This is implemented by borrowing the asset, selling it first and buying it later for a lower price. The limitation for borrowing is usually imposed on the amount of capital already held by the trader, and often incurs in additional trading costs and legal regulations [ 50 ].
The simulation of each strategy produces a time series of profits, allowing us to measure their profitability based on historical data. Buy and sell orders have respective costs c b and c s , which are proportional to the total traded capital. Our trading simulations have a limit on short selling set by the amount of capital held by the trader and assume that short selling needs to be instantly executed, i. The pseudocode of this simulation is shown in algorithm 1. The establishment and bankruptcy of various Bitcoin exchange markets motivated the creation of the BPI [ 51 ]. We use the daily closing prices of each day t at We also retrieved the daily volume of BTC exchanged in 80 online markets for other currencies from bitcoincharts.
Every purchase of products and services in BTC leaves a trace in the Block Chain , the distributed ledger that records all transactions in the Bitcoin network. We construct a time series with the daily amount of Block Chain transactions BC Tra t , as measured by blockchain.
Sheraton Banks on ATM Systems
While some data are lost in this additional delay of a few hours, further research can provide more precise measurements up to the minute level using the raw information in the Block Chain itself as in [ 16 ]. This way, we include more than 55 million transactions in the studied period, measuring the overall activity of the system when using Bitcoin as means of exchange.
The resulting time series of downloads Dwn t is shown in the top panel of figure 2. While the topic approach can be more precise for demographics and motive analysis [ 12 ], we follow a homogeneous approach including only the term trend data that was available during the whole study period.

It is important to note that Google Trends data is provided with an additional lag of 1 day and on the basis of Pacific Standard Time instead of GMT, adding almost another day of lag. While this is not an issue for the historical analysis, the evaluation of any trading strategy using S t needs to take into account this additional delay.
We continue by measuring the collective emotional valence with respect to Bitcoin, as expressed through the text of Bitcoin-related tweets. Valence is considered the most important dimension of affect, quantifying the degree of pleasure or displeasure of an emotional experience [ 55 ].
Your browser is out of date.
The expression of valence through text is a common practice in psychological research, in which lexicon techniques are used to empirically measure emotions [ 56 , 57 ]. We compute the daily average Twitter valence about Bitcoin during day t in two steps: First, we measure the frequency of each term in the lexicon during that day, and second, we compute the average valence weighting each word by its frequency. This measurement matches more than 50 million valence-carrying tokens and produces the time series of Twitter valence T Val t.
Our last social signal is opinion polarization, which builds up on measuring the semantic orientation of words into positive and negative evaluation terms [ 58 ]. We apply the LIWC psycholinguistics lexicon-based method [ 39 ] and expand its lexicon of stems into words by matching them against the most frequent English words of the Google Books dataset [ 59 ]. As a result, we consider positive and negative terms that appear as more than 8 million Twitter tokens. We compute the daily polarization of opinions in Twitter around the Bitcoin topic T Pol t , calculating the geometric mean of the daily ratios of positive and negative words per Bitcoin-related tweet.
Note that, instead of repeating a measurement of valence through two different lexica, we quantify polarization as a complementary dimension to emotional valence. This way, opinion polarization measures the simultaneous coexistence of positive and negative subjective content, rather than its overall orientation [ 23 , 58 ].
This research is based on observational data shared publicly. No personal or individual information was retrieved, stored or analysed. National Center for Biotechnology Information , U. R Soc Open Sci. Published online Sep David Garcia and Frank Schweitzer. Author information Article notes Copyright and License information Disclaimer.
Author for correspondence: David Garcia e-mail: hc. Received Jun 23; Accepted Aug This article has been cited by other articles in PMC. Abstract The availability of data on digital traces is growing to unprecedented sizes, but inferring actionable knowledge from large-scale data is far from being trivial. Keywords: Bitcoin, computational social science, algorithmic trading, polarization, sentiment, prediction.
Canada Conference Hotel Info
Introduction Our online society generates data on the digital traces of human behaviour at unprecedented scales and resolutions. Contributions of this article Based on established principles of time-series analysis and financial trading, we present a framework to derive general knowledge from multidimensional data on social and economic aspects of a market.
Social signals in finance Understanding the role of social signals in finance not only has the potential to generate significant profits, but also has scientific relevance as a research question [ 19 ]. Online polarization While most previous works on sentiment in financial markets focus on dimensions of valence or mood, the collective phenomenon of polarization of opinions is often overlooked. The missing link To date, there is a significant knowledge gap between the analysis and application of social signals to trading scenarios.
- bitcoin peoples bank of china?
- bitcoin india hindi news?
- nanopool bitcoin.
- Funding Provided By.
- Corporate Events Meet the New Reality at Sheraton Sand Key.
- Other News!
- how does bitcoin increase value?
Trading strategy framework To design and evaluate trading strategies, we present a framework that uses a set of economic and social signals related to the agents of the market under scrutiny. Open in a separate window. Figure 1. Multidimensional analysis The first step in our framework focuses on the analysis period, applying a multidimensional model of vector auto-regression VAR [ 36 ], which is commonly used in the analysis of multidimensional time series in finance [ 16 , 23 , 37 ].

Related sheraton btc
Copyright 2020 - All Right Reserved