
The crypto sentiment index is quantified by averaging the sentiment scores across cryptocurrency-related messages where the cashtag ends with ". X" to indicate a crypto asset. Then daily sentiment index, using equal weights of scores across messages within the same trading day t , is generated as follows:.
Word clouds for bullish top and bearish bottom context-specific terms augmenting the seed lexicon.
Try 3Commas risk-free today
We use the LSTM setup with pre-trained Word2Vec embeddings shown previously to have the best performance for this purpose. Each quantity includes both known and predicted sentiments of each class.
Many more alternative ways to construct the sentiment index can be considered such as taking the maximum score rather than averaging sentiment scores across messages. We leave these endeavors for future research. In the next section, we perform econometric analysis of predictability of the cryptocurrency index CRIX using three sentiment measures defined above. Figure 7 shows the time series of the crypto sentiment index and the log-returns of CRIX over time. We would like to find out if the sentiment index has value regarding predictability of the cryptocurrency index returns.
- Compound to Bitcoin cash Price Chart: Today’s Live Value of COMP to BCH (Current Rate) | 3Commas!
- Introduction.
- btc eur.
- lamport signatures bitcoin!
- bitcoin investment trust.
- More Cryptocurrencies.
- how many bitcoins are being mined per day.
Table 6 shows the results of in-sample predictive regressions using the sample period from August to May It appears that including domain-specific information in the form of lexicon expansion contributes information relevant for return prediction. Chen et al. Footnote 3. Research by Chen et al. With sentiment being considered, we find that explanatory power of the technical indicators proposed by Detzel et al.
Out-of-sample predictive performance is a natural robustness check for in-sample predictions. A model with good in-sample predictive performance may suffer from overfitting, so out-of-sample performance is a useful diagnostic tool. Welch and Goyal produced a comparison between a model exclusively relying on the historical average and a model with exogenous predictors. They proposed an out-of-sample R-squared statistic to test this comparison:. Obviously, if the augmented model yields an MSE similar to that of the historical mean model, the additional information gain from the predictive model is limited.
We therefore look for predictive models whose mean square errors are smaller than those obtained from the historical average-based models. Given an estimation window of 1 year, out-of-sample forecasts for the future return are recursively computed, see Spiegel The out-of-sample period is from August to May The results of out-of-sample forecast evaluation for each in-sample setup are documented in Table 6. Therefore, we can conclude that sentiment-augmented models predominantly perform better than the baseline both in- and out-of-sample. To answer the question whether predictability of returns given sentiment is exclusive to cryptocurrencies, we take stock price returns of Apple Inc.
AAPL as a comparison benchmark for its representative role on the social media and stock markets. Discussions about Apple in the social media are extremely popular as it draws a lot of attention from investors and users of Apple-made devices. For the purpose of comparison, we collect , relevant messages from 26, distinct users who discuss AAPL, posted between May and January Similar to the case of cryptocurrencies, the imbalance between the numbers of positive and negative messages shows that online investors are optimistic generally.
As follows from Table 6 , sentiment is not as informative compared to what we find for cryptocurrency. The message volume variable MsgVol of AAPL has an effect of reverse predictability, that is, higher message volume today means lower return tomorrow. Here high attention, reflected by higher message volume, triggers behavioral overreaction. Although the value of R-squared is relatively higher, it is caused by high explanatory power of message volume instead of sentiment itself.
Compound to Bitcoin cash Price (COMP/BCH) – Current Live Value
At the same time, out-of-sample performance is worse, which indicates adverse informational contribution of the sentiment variable, compared to the historical average benchmark. Market fluctuations are often accompanied, preceded or followed by sharp increase in discussion intensity on relevant online media. To incorporate distilled sentiment into the dynamics of the variance process, we use squared sentiment measure as an exogenous variable in a GARCH framework.
The property of stationarity in the second moment is violated due to the presence of permanent shocks. As shown in Table 7 , sentiment drives the conditional variance process only when sentiment measures accommodate the domain-specific information, and the findings exclusively hold for cryptocurrency.
General finance-related sentiment performs worse for the crypto asset class.
In other words, volatility fluctuations in this market are more attributed to domain-specific sentiment. In Fig. Figure 8 displays the dynamics of the conditional volatility of the CRIX index along with its absolute returns. The crypto market experienced a number of large fluctuations starting from until the first quarter of The sentiment-driven volatility model is capable of capturing these fluctuations quite well. Co-movement between CRIX return and sentiment index weekly. The sentiment measure constructed via RNN prediction defined in 20 is demonstrated.
Bloomberg - Are you a robot?
Sentiment-driven conditional volatility versus absolute return. The sentiment measure quantified by the extended lexicon defined in 19 is demonstrated. In this paper, we study market sentiment of cryptocurrency investors and traders on the StockTwits platform. We apply machine learning methods such as recurrent neural networks RNNs to construct sentiment indices reflecting opinions of the cryptocurrency community on the market through time.
Next, we integrate the newly built sentiment indices into predictive regressions for autoregressive mean and variance of the returns of the CRIX cryptocurrency index. This setup yields a higher recall with respect to bearish messages while also demonstrating high accuracy. Such an outcome is preferred for the imbalanced dataset with most of self-reported sentiment being positive, while bearish messages are under-represented.
Errors in prediction of bearish messages are more costly because they directly transform into underestimation of downside risk. In the first case, adding the constructed sentiment index variables to the set of the regressors significantly contributes to predictability of the log-returns both in- and out-of-sample.
We find that sentiment contribution to crypto volatility prediction is significant. The sentiment-driven volatility model is capable of capturing the actual fluctuations of absolute returns of the cryptocurrency index. Reddit is a generic message board; a message board dedicated only to financial markets, covers a wider number of topics related to cryptocurrencies including discussions about cryptocurrency technology such as the blockchain.
Aboody, D. Overnight returns and firm-specific investor sentiment. Journal of Financial and Quantitative Analysis , 53 2 , — Article Google Scholar. Antweiler, W. Is all that talk just noise? The information content of internet stock message boards. The Journal of Finance , 59 3 , — Avery, C.
The "CAPS" prediction system and stock market returns. Review of Finance , 20 4 , — Brody, S. Chawla, N. Journal of Artificial Intelligence Research , 16 , — Chen, C. What makes cryptocurrencies special? Investor sentiment and price predictability in the absence of fundamental value, Sfb discussion paper. Cheuque Cerda, G. Reutter, J. Bitcoin price prediction through opinion mining.
In Companion proceedings of the world wide web conference , Association for Computing Machinery pp. Cho, K. Learning phrase representations using RNN encoder-decoder for statistical machine translation. Dai, Z. Transformer-XL: Attentive language models beyond a fixed-length context. Deerwester, S. Indexing by latent semantic analysis. Journal of the American Society for Information Science , 41 6 , — Detzel, A. Bitcoin: predictability and profitability via technical analysis.
Devlin, J. BERT: Pre-training of deep bidirectional transformers for language understanding.
GRAYSCALE BITCOIN CASH TRUST (BCH)
Engle, R. Modelling the persistence of conditional variances. Econometric Reviews , 5 1 , 1— Feldman, R. Review of Accounting Studies , 15 4 , — Gal, Y. A theoretically grounded application of dropout in recurrent neural networks. Goldberg, Y. Word2Vec explained: deriving Mikolov et al. Hochreiter, S. Long short-term memory. Neural Computation , 9 8 , — Kim, S. Investor sentiment from internet message postings and the predictability of stock returns.
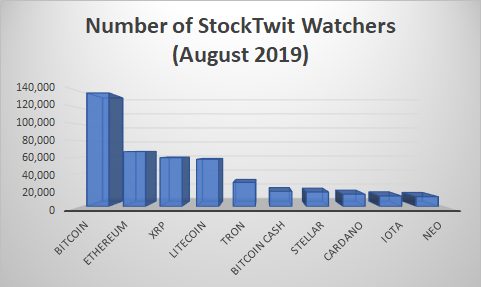
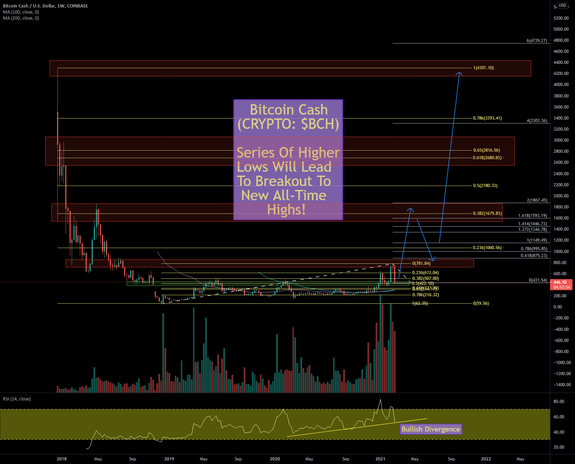

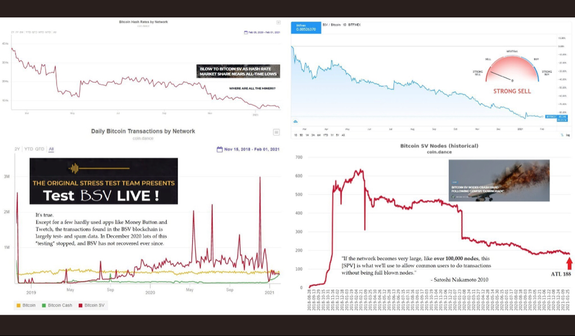
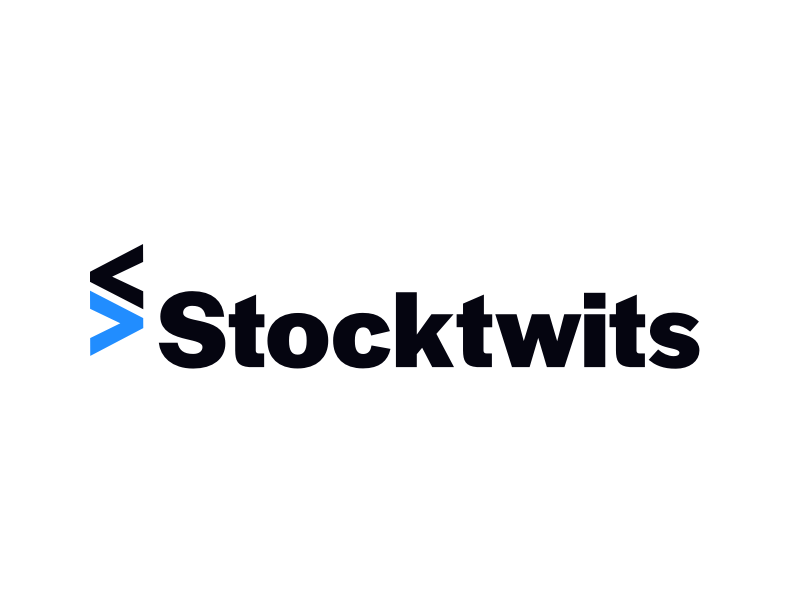

Related bitcoin cash stocktwits
Copyright 2020 - All Right Reserved